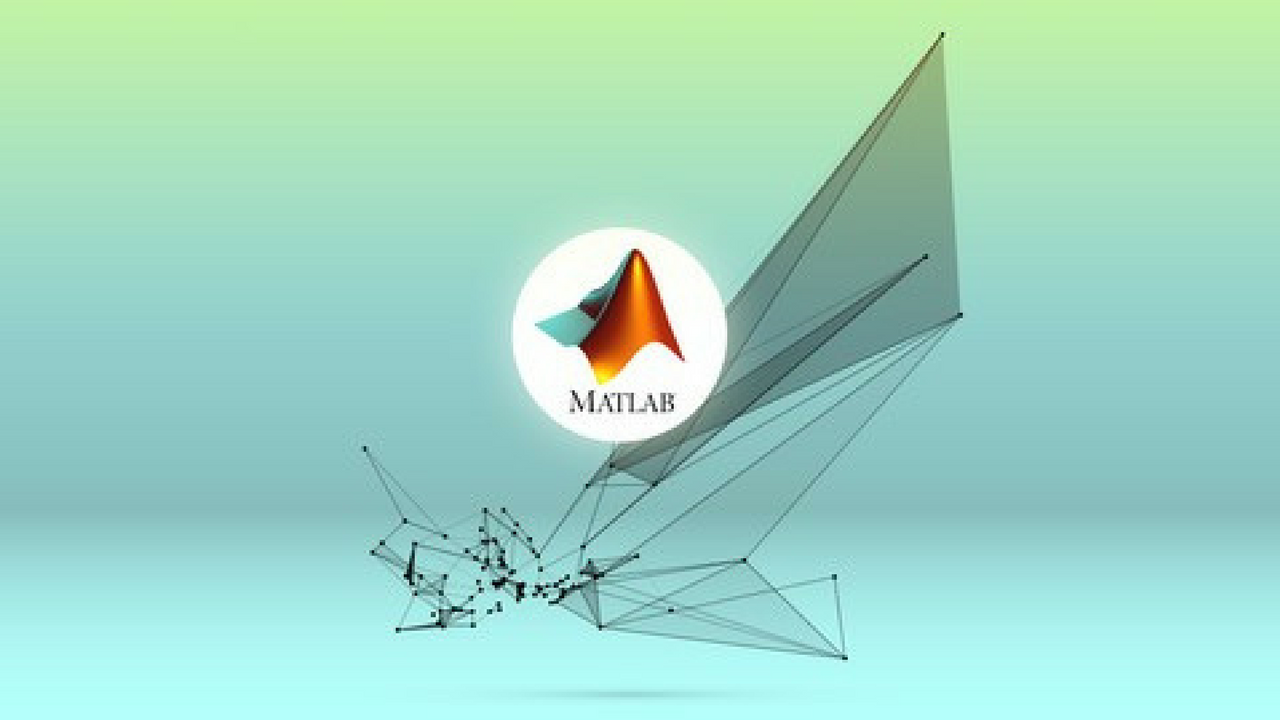
In this lecture we’re going to talk more about the Gaussian distribution. So the first thing we’ll ask is we’ve generated data so far from uniform distributions, how can we generate data for random normal distributions? So there’s a function in matlab called randn that will do this. So if you type in randn it should give you different function signatures. So let’s say we want to generate a thousand random normal values. Okay, and so this can represent any number of random random values. So if you download data from Kaggle or some other data set where you want to do machine learning or statistics on, you want to know the distribution of your input. In this case, we want to know if the distribution is normal or not. So one thing we could do to check that is just to plot it, plot the histogram. Alright, so, you can see that it looks relatively normal. We can use more bins to get a finer granularity. So we still see this same type of distribution even with 50 bins, and of course if we increase n our curve looks closer and closer to the Gaussian curve that we saw from the last lecture. Now of course in the real world your data is not going to look this nice and clean since your distribution may or may not be Gaussian distributed. The question now is how do you test if your data is Gaussian distributed or not? So we’re going to talk a little bit about hypothesis testing, but not too much since it’s a pretty long and difficult subject. So the basic idea with hypothesis testing is you have two different hypotheses. So in this lecture we’re not going to talk about the mechanics behind statistical testing, we’re going to kind of work backwards and jump right into statistical tests that you can use in matlab right away. So the first is a Jarque-Bera test. In matlab it’s the function jbtest. So you can use it to return a hypothesis and a p-value. The hypothesis will be whether or not your data is randomly distributed, and it returns a p-value to tell you the strength of that hypothesis. So let’s try this on our data that we just generated. Alright, so, one thing that we need to talk about is how to interpret the return values of the Jarque-Bera test. So, when we talk about hypothesis testing you’ll see that there is a null hypothesis and an alternative hypothesis. The Jarque-Bera test will return one if we reject the null hypothesis, and the null hypothesis is that our data is normally distributed. So if we pass it in R, we get the hypothesis is the null hypothesis and a p-value of .5 which means we do not reject the null hypothesis that our data is normally distributed. There is another test called the Kolmogorov-Smirnov test which essentially does the same thing. So the null hypothesis again is that the data comes from a normal distribution, and the result h will be 1 if you reject the null hypothesis, so let’s try that. Same thing with kstest, right, and so this also rejects or does not reject the null hypothesis that the data is normally distributed. So now let’s do something interesting, let’s generate some random data that we know is not normally distributed. So, we’ll do this data from a uniform distribution. So now I want to do jbtest on uniform data right. So we get h equals 1 which means we do reject the null hypothesis, and our p-value is one one-thousandth, and usually a p value is determined significant when it’s less than five percent. So, what this is basically saying is that our uniform generated data is not Gaussian distributed which we know already is true. We can do the same test with Kolmogorov-Smirnov, and so this also rejects the null hypothesis with a much smaller p-value. Alright, and so this is how you can test if your data is normally distributed or not.