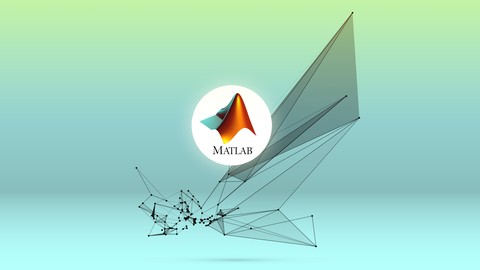
Image processing in MATLAB
So, in this matlab tutorial we’re going to switch gears a little bit to a related topic image processing. The first thing I want to talk about is what is an image? So, we can compare it to soundwaves since we’ve already studied those. Now let’s think about the most raw data possible. So, firstly, whereas sound is a one dimensional function, images are two dimensional functions. We have an x, a y, and a z, and so that z is the value at that x and y. In fact, you can think of a heat map or a contour map as basically images. So, we have a 2d function, ok, x & y are continuous coordinates in space. And like with sound we have to sample the data in order to store it in a computer. So, with sound we’re sampling across time and we call that the sampling frequency. What’s analogous to the sampling frequency when we’re dealing with images? I’ll give you some time to think about that, this is actually probably pretty familiar to you. So, with images the analogous concept would be the resolution, right, so the amount of fine-grainess you put into each point in space. For example, Udemy videos are required to be recorded in HD resolution. So, now let’s suppose we have an image called a, a will have the indexes i and j referring to be xy coordinates. Just write this down if it helps you. So, we have an image a, and then a(i,j) would be the xy coordinates. So, now let’s think about what would be stored at a(i,j). In other words, how do we store a pixel? So, now let’s suppose we store just one number at a(i,j), so it’s a two-dimensional matrix or a table. A table of values at each point xy. That image would be, that matrix would represent a grayscale image, right, because if we only have one number we can only represent intensity. So, 0 would be black and 1 would be white, and so a(i,j) would be the intensity at the point ij. In order to store a color we need three parameters r, g, b, or red, green, and blue, and this is what we’ve been looking at for the entirety of this video so far. So, these values usually vary from 0 to 255 so we can have a total of 256 to the power of 3 possible different colors. So, let’s look at what the value of that is. So, that’s about 16.8 million different colors we can represent if we store values from 0 to 255 for RGB. Now what this also means is that if we have a 500 x 500 image, the matrix that we use to represent it isn’t 500 x 500 but it’s 500 x 500 x 3. Sometimes this is called an image bitmap. Alright, so now that we’ve talked about images in the abstract sense, let’s talk about how you would actually open an image in matlab. There’s an image processing tool box that we won’t be using a lot of in these tutorials. Matlab has a function called imread built-in. We’re going to use that function to read in a very famous picture often used in image processing, so if you ever take a computer vision course or multimedia course you’ve probably seen this image before. Ok, so, let’s look at the size of a. So, it’s 512 x 512 x 3, so a three dimensional matrix as promised. Notice the data type is uint8 instead of double that I have for my other matrices which is the default in matlab. So, a unint8 the u stands for unsigned, int stands for integer, and eight means it’s 8-bit. Ok, so, we think about how many values could be stored in an unsigned 8-bit integer let’s go ahead and calculate that. You should pause this video and try to think about the answer yourself first. Ok, so, the answer is 2 to the power of 8, and that’s 256. So, as we discussed previously values for R, G, and B go from 0 to 255 which is 256 different values. There’s a function called imshow that will essentially plot the matrix as an image. So, that’s the image, the famous Lena image, that’s used for image processing.